Understanding the effects of presenter gender, video format, threading, and moderation on YouTube TED talk comments
Scholars, educators, and students are increasingly encouraged to participate in online spaces. While the current literature highlights the potential positive outcomes of such participation, little research exists on the sentiment that these individuals may face online and on the factors that may lead some people to face different types of sentiment than others. To investigate these issues, we examined the strength of positive and negative sentiment expressed in response to TEDx and TED-Ed talks posted on YouTube (n = 655), the effect of several variables on comment and reply sentiment (n = 774,939), and the projected effects that sentiment-based moderation would have had on posted content. We found that most comments and replies were neutral in nature and some topics were more likely than others to elicit positive or negative sentiment. Videos of male presenters showed greater neutrality, while videos of female presenters saw significantly greater positive and negative polarity in replies. Animations neutralized both the negativity and positivity of replies at a very high rate. Gender and video format influenced the sentiment of replies and not just the initial comments that were directed toward the video. Finally, we found that using sentiment as a way to moderate offensive content would have a significant effect on non-offensive content. These findings have far-reaching implications for social media platforms and for those who encourage or prepare students and scholars to participate online.
Paradigmatic Analysis
This mixed methods study primarily operated from a positivist paradigm and used quantitative analysis via structural equation modeling (SEM) to determine the effects that presenter gender of YouTube informational videos had on comment sentiment. The study also lightly used qualitative elements by relying upon human coders to code comments in accordance with a priori sentiment codes, but the overall tenor of the article nonetheless remains highly positivist in nature, because it assumes objectivity, assigns a priori numeric values to phenomena being studied, makes predictive claims, and makes claims of generalizability based upon randomization, representative sampling, and significance testing.
Introduction
Public online spaces, such as social media, have often been promoted as places that are fit for teaching and learning. From Jenkin’s work on participatory cultures (Jenkins, 2006a, 2006b) to online learning approaches founded upon networked learning principles (Goodyear, 2005; Steeples, Jones, & Goodyear, 2002), to scholars’ efforts to engage large and diverse publics via networked technologies (Veletsianos & Kimmons, 2012), the public Web has often been characterized and envisioned as a democratizing space that could foster interaction, collaboration, and debate (Shirky, 2008; Van Dijk, 2012). These positive aspects of the Web however, are neither guaranteed nor established in all contexts. Recent research demonstrates that public online spaces may reinforce social stratification, yielding unequal benefits for different participants (Huang, Cotten, & Rikard, 2017; Robinson et al., 2015; Yates, Kirby, & Lockley, 2015), foster abuse and harassment (Duggan, 2014; Ybarra & Mitchell, 2008; Winkelman, Early, Walker, Chu, & Yick-Flanagan, 2015), limit diversity of opinion and conversation by encouraging echo chambers (Flaxman, Goel, & Rao, 2013, 2016), and in general reflect and reinforce sociocultural biases.
The literature on the educational uses of the public Web focuses largely on ways to design or use online media to engender more positive learning experiences (see Conole & Alevizou, 2010; Tess, 2013). Nevertheless, as the public Web begins to permeate every aspect of scholarly activity, as students are asked to participate in online communities or as scholars are encouraged to “go online,” create a digital identity, and expand the impact and reach of their scholarship (Lowenthal, Dunlap, & Stitson, 2016; Watters, 2016; Weller, 2011), we need to be cognizant of the fact that emerging research suggests that negative reactions, harassment, and incivility feature prominently on the Web.
This study therefore, was developed to gain a better understanding of the sentiment that educators and scholars may face in online contexts. Are educators and scholars exposed to negative sentiments when participating online? What variables might mediate their exposure to negativity? Does comment moderation curtail negativity? The results of prior research suggest that gender, video format, and comment moderation may have significant relationships to sentiment toward videos that scholars post online. To explore sentiment toward public scholarly content, we analyzed YouTube comments posted in response to TED-style talks. In particular, we ask what sentiment is expressed toward scholars, how the gender and delivery format influence expressed sentiment, and whether active comment moderation has any impact on overall sentiment expressed. Following a review of the literature relevant to this topic, we describe the methods used, present our findings, and conclude with a discussion of these findings in the context of current literature.
Literature Review
Incivility happens everywhere. Thus, we are not surprised to find incivility online. Using the public Web, and specifically social media, for educational purposes can expose teachers and students to unwanted, rude, harassing, or, in general, unsociable behavior. However, educators are traditionally not used to incivility in the classroom (see also Bjorklund & Rehling, 2009). Thus, we are interested in how sentiment, and especially incivility, manifests itself on the public Web in educational spaces. Building upon the work of Tsuo, Thelwall, Mongeon, and Sugimoto (2015) who investigated sentiment expressed in comments toward a sample of TED talks, we conducted a census study of the sentiment in the comments of TED-type videos posted on YouTube. In the following section, we summarize the literature on sentiment and incivility to ground our study.
Sentiment
Ortigosa, Martín, and Carro (2014) defined sentiment simply as “‘a personal positive or negative feeling or opinion”’ (p. 528). Sentiment is a fact of life; everyone has feelings and opinions. These feelings influence what people say and how they say it and in turn how people communicate and interact, whether online or face-to-face. However, online communication--which is still primarily text-based--differs from face-to-face communication in many ways. For instance, online communication lacks the visual and nonverbal cues that people use to express sentiment. Thus, when communicating online, phrasing, terminology, and use of capital letters/symbols all contribute to the underlying meaning of text and the mood or personal state of the author (Bollen, Mao, & Pepe, 2011). Sentiment is important because it can convey deeper meaning, revealing positive and negative aspects of opinions (Pang & Lee, 2008), personal levels of happiness or sadness (Dodds & Danforth, 2010), or even emotional health (Kramer, 2010).
Sentiment stems from personal emotion and motivation, drawing upon perspective, experience, persona, and more. However, external factors such as political, cultural, and economic events influence and correlate with sentiment (Bollen, Mao, & Pepe, 2011; Thelwall, Buckley, & Paltoglou, 2012). When trending topics involve tragedy, sentiment can range from sorrow to outrage. When trending topics involve national holidays, sentiment can range from happiness to patriotism. As the world increasingly turns to the Web for a variety of social interaction, sentiment takes on a larger role in terms of how and why we engage or disengage.
However, unlike in the past, people’s daily use of the public Web, and specifically social media, puts their feelings and opinions--that is, their sentiment--on display for the world to see. This in turn has sparked new interest in sentiment analysis or opinion mining, which Medhat, Hassan, and Korashy (2014) explain is “the computational study of people’s opinions, attitudes and emotions toward an entity” (p. 1093). Early research using sentiment focused mostly on understanding public sentiment on politics (see Rowe, 2015) or products/services (see Turney, 2002; Pang, Lee, & Valithyanathan, 2002). However, researchers have begun to use sentiment analysis to better understand the nuances of online communication, to include impacts on interaction, effects of negativity and trolling, how gender factors into interaction, and the underlying causes of these behaviors. Cambria, Schuller, Xia, and Havasi (2013) argued that “although commonly used interchangeably to denote the same field of study, opinion mining and sentiment analysis actually focus on polarity and emotion recognition, respectively. Because the identification of sentiment is often exploited for detecting polarity; however, the two fields are usually combined under the same umbrella or even used as synonyms” (p. 15). This combination creates a rich foundation upon which research and conversations surrounding online discourse may occur.
Format
The question of format or platform emerges as another component to the sentiment and online communication phenomenon. In other words, do different formats; e.g., YouTube or Instagram, or platforms of sharing; e.g., news media or social media, invite different sentiment or encounter more comments of negativity in public responses? Given the relative infancy of this line of inquiry, emerging research gives us little insight into what we might expect. For example, Ksiazek, Peer, and Lessard (2016) found that, “viewers of online news videos are more likely to engage in user–content interaction for popular videos, but are more likely to engage in user–user interaction with less popular ones” (p. 513). The researchers further noted that public measures, such as liking, commenting, and sharing, contribute to this definition of popularity and sometimes do not reflect quality of the media content. In this respect, content may be more likely to trend or appear in aggregated platforms thereby calling attention to the source and generating more comments based solely on the headline or title, thumbnail image, or existing comments. Walther, Deandrea, Kim, & Anthony (2010) partially explained this aspect of online communication as reactive rather than interactive, cautioning that platform does contribute to the situation. Consider the difference between comments on a video uploaded directly to YouTube and a link to this video shared on Facebook or Twitter. Activity on the uploaded media generally trends towards direct comments about the video, including sentiment about the speaker and/or topic. Rarely do comments represent a dialogue emerging of comments between users responding to one another. Looking at the comments on a Facebook post that links to this same video reveals a distinctly different trend. That is, comments may lead to nested replies wherein an interactive conversation emerges. Unfortunately, scholarly studies of this behavior to not necessarily examine why or how it occurs, only that it does and general characteristics of the phenomenon. Thus, we must look for other areas of inquiry that may help explain or refine our questions regarding sentiment and comments.
Toxicity in Threads or Sequential Sentiment
To help explore toxicity and sentiment in discussions, researchers have drawn upon a variety of communication, psychological, and educational theories in the place of a unified or singular theoretical foundation. The masked identity phenomenon illustrates how the anonymity of many online interactions increases participation while simultaneously providing an avenue for aggression and negativity (Ferganchick-Neufang, 1998). In other words, using pseudonyms or impersonal screen names allows for users to interact free of fear from retribution or personal information being divulged. This freedom empowers some users to speak more candidly than they might otherwise do in person or if his or her identity were known. As these users communicate with aggression; i.e., swearing or threatening, we see a contagious contamination effect whereby this aggressive behavior spreads to other users (Kwon & Gruzd, 2017). The festering nature of negativity in online communication sentiment takes on a dynamic and even cancerous-like effect. Indeed, Cheng, Bernstein, Danescu-Niculescu-Mizil, and Leskovec (2017) also concluded from their study that an individual's mood as well as the surrounding context of an online discussion can trigger anyone to engage in trolling or assume aggressive online behavior. Given the ubiquitous use of online communication, particularly for educational purposes, these findings and common behaviors represent a problematic challenge. Despite the propensity for negativity and incivility to prevail, Han and Brazeal (2015) set out to prove the power of civility in online discourse. In their study of political discussions in particular, the researchers based their hypotheses on the probability that collective incivil behavior of a group breeds incivil behavior among individuals within that group, demonstrating the power of modeling behavior. Despite examining the issue from very different fields with varying methods, all of these studies paint a dismal picture of online interaction and call to question ways in which incivil behavior has been mitigated.
Moderation
Comment moderation has been proposed as one way to safeguard against toxic comments online and curtail abuse. In light of increasingly negative sentiments left in comments on news articles and videos, including overt and covert racist, misogynistic, homophobic, and xenophobic statements, multiple outlets have been forced to address the concept of moderation. In general such decisions range from the absolute (e.g., eliminating the ability to comment altogether) to the relative (e.g., only allowing registered users to comment, only allowing users with past approved comments to post comments, or approving all comments prior to posting) (see Harlow 2015; Muddiman & Stroud 2017). If commenting is allowed, users most often must register and provide “real life” contact information about their identities, ways to verify their identity, and how they may be contacted if need be. When the Buffalo News eliminated anonymous commenting in 2010, a debate erupted regarding privacy concerns as well as the probability of such policies impacting First Amendment rights to free speech (Hughey & Daniels, 2013). Indeed, anonymity often represents the ability to freely and openly express one’s opinion, free of retaliation or resentment (Reader, 2012). However, in the wake of the moderation revolution, an interesting trend has emerged. While seeking categorize user-generated content in participatory journalism, Ruiz et al. (2011) found that moderated comments lead to two models of participation; communities of debate that engage in primarily respectful discussions about the topic and homogenous communities that express more personal feelings with little interaction among users. These findings are predicated on the fact that inflammatory and/or incivil comments that might incite discord or argument are not allowed into the conversation, effectively censoring some users. Though outside the scope of this study, it might be interesting to investigate how to moderate comments in a way that prevents ongoing negativity but that also does not unduly censor the community. Nonetheless, comment moderation or the removing the ability to comment on media at the very least becomes a significant decision for educators to make when either producing or instructing students in producing online media.
Gender
The last consideration in the context of sentiment and civility involves the role of gender, both as the subject or target of online content and as a user who comments on content. Incidents of online incivility and how gender contributes to the situation have gained increasing research attention in recent years. The Pew Research Centre (Duggan, 2014) and the Data & Society Research Institute (Lenhart, Ybarra, Zickuhr, & Price-Feeney, 2016) conducted two large-scale surveys in the United States and both reported that over 40% of surveyed Internet users have experienced some form of online abuse. Both of these studies found that a person’s gender mediates the type of toxic comments directed at them, with women reporting more severe and sustained forms of abuse. Women, and younger women in particular, are more likely to experience a wide variety of abuse or harassment, to include purposeful embarrassment, being called offensive names, physical threats, sexual harassment, and even being stalked (Lenhart, Ybarra, Zickuhr, & Price-Feeney, 2016). Returning the conversation to education in particular, gender already sits atop the list of issues for attention. In a survey of faculty members at one Canadian university, Cassidy, Faucher, and Jackson (2014) showed that female faculty members reported a higher level of online harassment (22% reported being harassed in the past year compared to 6% of males), and were much more likely to complete the survey and volunteer for interviews. Participants reported that some of this harassment occurred through social media, and this finding provides a rationale for specifically studying the ways that gender may mediate sentiment. Women experience incivility and harassment across social media, online discussion forums, and multiplayer video games; leading to a conclusion that sentiments directed towards women are not exclusive to any one format, technology, or platform. Thus, this study should account for the overwhelming likelihood for women to encounter direct and indirect incivility online.
Theoretical Framework
This study is situated on the Networked Participatory Scholarship (NPS) framework. NPS refers to scholars’ presence and use of “online social networks to share, reflect upon, critique, improve, validate, and otherwise develop their scholarship” (Veletsianos & Kimmons, 2012, p. 768). NPS is an appropriate framework for this investigation because it is non-deterministic and agnostic. In other words, it does not position scholars’ online presence as having an inherently positive or negative aspect to it. Under this framework, participation in online spaces (e.g., posting of videos and online comments/replies) becomes a situated activity of facilitation, discussion, negotiation, and co-construction of knowledge (cf. Brown, Collins, & Duguid, 1989; Lave & Wenger, 1991; Wenger, 1998). The framework emphasizes that digital technology is influenced by social, technological, cultural, economic, and political factors, and as such, a wide array of forces will encourage, restrict, and impact digital comments. In addition to the effect of the actual platform studied (i.e. YouTube), other forces that we anticipate will impact expressed sentiment and comments may include speakers’ gender, delivery format, and comments made by other participants. This perspective aligns with the social construction of technology theory (Pinch & Bijker, 1984), which suggests that individuals’ actions shape the ways that a particular technology is used.
Furthermore, we theorize that due to the fact that gender norms are policed in Western society in mainly discursive ways (Butler, 1990), YouTube comments/replies may be used to silence, threaten, or otherwise harass female academics (Baym & boyd, 2012; Jane, 2017; Taylor, 2012). Therefore, we expect the sentiment expressed toward female presenters to be more negative than toward male presenters. We anticipate that the absence of visible gender (in the form of video animations) will reduce this phenomenon, but it’s also likely that the presenters’ voice will act as a gender cue such that gender effects remain present even after the removal of presenters’ image (cf. Reeves & Nass, 1996).
Research Questions
To address the identified gaps in the literature, we posed the following research questions:
RQ1. How does the online community react to TED Talks on YouTube (in terms of comment sentiment)?
RQ2. How does the gender of the video presenter, the delivery format (presentation vs. animation), and comment threading influence the sentiment of comments and subsequent replies?
RQ3. What would be the projected effects of moderating negative comments upon community participation?
Context
To examine user sentiment toward public lectures we focused our efforts on the videos and comments posted in the TEDx and TED-Ed YouTube channels. YouTube is one of the most visited websites in the world (Snelson, 2011; Tsou et al., 2014) and, as such provides an authentic environment for natural experiments intended to study various aspects of online participation. For the purposes of this research, YouTube provides an environment to study how people communicate and specifically express sentiment toward public intellectuals such as speakers. The TEDx and TED-Ed channels are managed by TED (Technology, Entertainment, Design), an organization that hosts conferences and posts videos of speakers online for broad consumption. The content of these two channels is diverse. The TEDx channel hosts video recordings of speakers presenting to live audiences at TEDx events, while the TED-Ed channel hosts videos in animated form. The TEDx channel started in June 2009 while the TED-Ed channel was launched in March 2011. Both channels feature large numbers of YouTube subscribers (at the time of writing, TEDx: ~7.5 million; TED-Ed: ~4.5 million) and video views (at the time of writing, TEDx: ~1.4 billion; TED-Ed: ~630 million).
The TEDx and TED-Ed channels are a shining example of public interest in educational content and public scholarship. The videos are created by experts and are of high quality, and they are frequently promoted for their educational value. For instance, researchers have encouraged educators to use TED talks in their courses (e.g., DaVia Rubenstein, 2012; Romanelli, Cain, & McNamara, 2014) , and the TED-Ed channel is specifically designed to be used in educational settings.
Methods
We used a combination of web extraction, data mining, quantitative, and qualitative methods to answer the research questions. We describe our data collection and data analysis methods next.
Data Collection
We used the YouTube API to collect all data from publicly available videos listed in the TEDx and TEDed channels. In total, data for 1,080 videos were collected from the TEDx and TEDed channels at a similar rate (570 for TEDx [or 52.8%] and 510 for TEDed [or 47.2%]; cf. Table 1). Videos were manually coded for presenter gender, format, and delivery language. If a presenter explicitly self-identified as a gender in the talk or used a gendered pronoun in the talk description, we used those self-identifications as the gender code; otherwise, we interpreted presenter gender from names and visual appearance.
Table 1. Descriptive results of video and comment counts
Format
|
Gender
|
Videos
|
Video Comments
|
Comment n Avg
|
Comment n SD
|
Raw
|
|
|
|
|
Talk
|
Female
|
169
|
95,593
|
565.64
|
1,664.94
|
Talk
|
Male
|
432
|
261,765
|
605.94
|
2,333.35
|
Animation
|
-
|
479
|
431,360
|
900.54
|
1,649.78
|
|
1,080
|
788,718
|
730.29
|
1,960.11
|
Included
|
|
|
|
|
Talk
|
Female
|
75
|
92,932
|
1,239.09
|
2,328.55
|
Talk
|
Male
|
177
|
250,647
|
1,416.08
|
3,484.31
|
Animation
|
-
|
413
|
431,360
|
1,044.45
|
1,733.89
|
|
|
655
|
774,939
|
1,165.32
|
2,395.08
|
All comments for each of these videos were then collected. Many videos (33.61%) did not return any comments, suggesting that either the video blocked comments or that it simply did not garner any interest from the community. Given that the minimum comment count for videos with more than zero comments was 25, we determined it most likely that zero comment counts reflected comment blocking on the video. As we considered comment counts as a binary variable of either commented or not commented, a small-to-moderate Pearson correlation was detected between format and commented, r = .375, p < .01, revealing that animations were more likely to be commented upon than talks (i.e., less likely to be blocked). However, there was no significant correlation between gender and commented. So, we concluded that comment blocking was more likely for talks than for animations but that this blocking did not vary significantly by the gender of the presenter.
Excluding videos with comment counts of zero, the range of comment counts was 25 to 31,622, M = 1,100.03, SD = 2,319.57, revealing strongly positive skew and large variation. A series of non-parametric Mann-Whitney U Tests indicated that comment counts (1) did not vary by gender but that (2) they were slightly higher for animations (Mdn = 470) than for presentations (Mdn = 390), U = 56,953.5, p = .034, and (3) were much higher for English videos (Mdn = 467) than for non-English videos (Mdn = 167.5), U = 8,134, p = .00. Thus, we concluded that the number of comments was not influenced by gender but that animations elicited more comments than presentations and that English videos elicited more comments than their non-English counterparts.
Comment Coding and Sentiment Analysis
To consider each research question, we examined (amongst other things) comment order in replies. However, YouTube has not always had the reply feature, which allows users to comment in response to other comments, and previously users might respond to other comments by using an @ symbol at the beginning of the comment. In the data, the first instances of replies occurred on 11/7/2013, and so we excluded all comments from this analysis that occurred prior to this time. This reduced the total size of the comments dataset by only 9.23% to n = 703,340. We then organized comments into two groups, and we will operationalize our word use hereafter to delineate them as follows: (1) comments, representing top-level comments in response to the video, and (2) replies, representing replies to comments posted by other users. Comments and replies were almost equally represented in the dataset, with 354,539 comments and 348,800 replies.
We then used the open source sentiment analysis tool SentiStrength (Thelwall, Buckley, & Paltoglou, 2012) to generate sentiment scores for all comments and replies in the dataset. This tool has been used in prior literature and studies report that its precision is similar to human-level accuracy (e.g., Oksanen et al., 2015). SentiStrength generated two separate sentiment scores for each data item: positivity and negativity. Positivity was measured on a 1 to 5 scale, with 1 being neutral and 5 being extremely positive. Negativity was measured on a -1 to -5 scale, with -1 being neutral and -5 being extremely negative. This separation of sentiment into two separate constructs is grounded in literature in psychology (Berrios, Totterdell, & Kellett, 2015) that proposes that sentiment is constructed by counterbalancing these two separate phenomena. This is why it is possible to experience both positive and negative emotions at the same time (mixed emotions).
Comments and replies varied by sentiment along each of the 5-point spectra. Some example comments and replies exhibiting varying levels of sentiment are provided in Table 2. As these examples show, sentiment is a difficult phenomenon to understand with a single factor, because some forms of human expression will convey complex messaging with both highly positive and highly negative sentiment at the same time (such as in the mixed polarity examples). For this reason, we kept negativity and positivity as separate variables for our analyses moving forward.
Table 2. Example comments and replies at varying sentiment levels
Text
|
Negativity
|
Positivity
|
Sentiment Interpretation
|
We actually wrote a paragraph from the Narcissis story. It was... PAINFUL!!!!
|
-5
|
1
|
Very Negative
|
plus she's religious af and eveytime I tell her to go see a therapist she says that God is the only one she'll talk to that why I fucking hate religions
|
-5
|
1
|
Very Negative
|
I have motion sickness. So, long distance journey can be unpleasant. ...
|
-3
|
1
|
Negative
|
So where is your scientific proof she is just out to sell books? ... If she is right then 99% of studies are flawed. That pesky placebo is such a problem, isn't it? ...
|
-3
|
1
|
Negative
|
It can be pretty annoying because the sperm cell and easily fertilize the egg all of the sudden. ... Fortunately, there are less than a hundred fumale in the world and they are only found in very few parts of South Africa. Then it's easy for their stomach to explode. ...
|
-3
|
3
|
Mixed Polarity
|
You must be joking. ... Besides that though constructed languages are a breed all their own and they, while less of a concern than natural languages in most cases, should not be ignored.
|
-3
|
3
|
Mixed Polarity
|
God I'm from germany and I fucking hate this techno trash... but this guy is absolutely amazing :D insane beatboxer and really funny comedian at the same time c:
|
-5
|
5
|
Extreme Mixed Polarity
|
YET, he fucking loved every inch of his life and had no regrets, he inspired people and died doing what he loves. Dumb cunt........losers hating on him or criticizing him doesn't matter as his life was perfect and fulfilled, he found life and his soul was intact. So, go get a life bitch...
|
-5
|
5
|
Extreme Mixed Polarity
|
This made me ask myself: Where am I going with my life?
|
-1
|
1
|
Neutral
|
How did you know the narrator's name? Does it say somewhere?
|
-1
|
1
|
Neutral
|
Mann I love these riddles
|
-1
|
3
|
Positive
|
Same lmao xD
|
-1
|
3
|
Positive
|
i really loved the closin analogy she's recited. loved her voice and smile it's influential
|
-1
|
5
|
Very Positive
|
it's not sad :) He knows and share with us what happiness love and hope are... As so many have no idea about
|
-1
|
5
|
Very Positive
|
Generally speaking, we interpreted sentiment of an item as being directed toward parent items. That is, comment sentiment was directed toward the video, and reply sentiment was directed toward the video and/or parent comment (within the context of the video). In some of our analyses, we also incorporated a lag of up to four replies to account for replies responding to one another within the same comment thread. This was intended to account for sentiment effects over time (e.g., a negative reply engendering more negative replies). Various statistical tests were employed to answer each research question, which we will explain more within each of the appropriate results sections below.
Analytical Strategy
In order to answer the question of how does the sentiment of comments, gender of presenter, and form of delivery influence subsequent replies, multiple regression in the framework of structural equation modeling (SEM) was employed. Multiple regression has the advantage of isolating unique contributions of variance of independent variables in the presence of other variables while the SEM framework has the ability to deal with missing data and the added flexibility to deal with relationships among independent variables. The assumptions that need to be met in order for the results of multiple regression to be valid are: (a) linearity of relations between the independent and dependent variables (correct functional form), (b) independence of observations, (c) normality of the residuals, (d) equality of variance across the parameter space, (e) no extreme multicollinearity between the independent variables, and (f) no missing data in the independent or dependent variables. The assumptions of linearity, normality, and equality of variance will be checked with a histogram of residuals and a residual plot. The independence assumption is expected to be violated as there are theoretical clustering effects both at the comment and presenter level. This will be accounted for by employing multilevel modeling (Luke, 2004). The SEM framework allows the independent variables to be correlated with each other thus relaxing the multicollinearity assumption (Wang & Wang, 2012). The SEM framework also deals with missing data through the full information maximum likelihood (FIML) technique that has been shown to be more robust to missingness than listwise deletion or mean imputation (Little & Rubin, 2014). All analyses were performed in the SEM program Mplus 7.4 simultaneously (Muthén & Muthén, 1998-2015).
Limitations and Delimitations
This study faces a number of limitations and delimitations. First, the results are bound by the context of the study, and may not apply to platforms other than YouTube (e.g., lectures posted on Facebook or a publicly available video-hosting site) or video types other than TED-style YouTube lectures (e.g., instructional videos, step-by-step tutorials, etc). Second, results may only apply to those presenters whose videos garner popularity or pass a certain view/comment threshold. Third, it is unclear whether TEDx and TED-Ed commenters are reflective of the larger YouTube community or of the online commenters in general. Finally, the research focuses on computational evaluations of expressed sentiment. Sentiment is a complicated construct. For example, an individual can reply in an ironic - and thus positive - manner to a negative sentiment and perpetuate negativity. We’ve taken a number of steps to address this issue, such as for example disaggregating comments and replies and focusing our research on comments as a means to understand sentiment toward video vis-a-vis sentiment toward others commenters. Nonetheless, to gain a deeper understanding of sentiment, harassment, and civility more robust qualitative methods are necessary in future research. Though these limitations and delimitations reduce the scope of the study, we do not believe that they pose significant threats to the validity and reliability of the results presented herein. Readers however should keep these in mind when interpreting the results and considering how those might apply in their own contexts.
Results
Participation in these videos tended to represent standalone commenting rather than ongoing participation by the same users. Most commenters only posted 1 comment (Mdn = 1, M = 2.04, SD = 4.83) on 1 video (Mdn = 1, M = 1.38, SD = 1.43). In fact, 92.8% of users only commented on 2 or fewer videos. If a commenter left 15 comments or posted on 7 or more videos, then they were in the top 1% of participants for commenting frequency. This revealed strongly positive skew in commenting and suggested that very few participants engaged in conversations about TED talk channel videos over a period of time but that most seemed to be drawn to a specific video or topic in a standalone manner. We will now proceed to provide results on sentiment of these comments, variables that influenced sentiment, and the projected effects that sentiment-based moderation would have had on content.
RQ1. Sentiment Toward YouTube TED Talks
Across the entire dataset, 0.6% of comments and 0.67% of replies were extremely negative. Conversely, 0.28% of comments and 0.1% of replies were extremely positive (cf. Table 3). Thus, both scales revealed that most comments and replies skewed toward neutrality (rather than polarity) in sentiment, with 63.48% of comments and 58.85% of replies exhibiting no negativity and 50.14% of comments and 57.48% of replies exhibiting no positivity. Depending upon where we set our sentiment expectations, this finding might mean very different things. A more calloused reader might, for instance, interpret this to mean that only a very small percentage of comments exhibited any form of negativity, while a more sensitive reader might interpret this to mean that one-third to one-half of comments were negative in some way. In either case, some level of negativity (e.g., disagreement) should be expected in any space where ideas are shared and explored, but extreme cases of negativity did not seem to be the norm in the dataset.
Table 3. Video top-level comment sentiment frequencies
|
Comments
|
Replies
|
|
n
|
354,539
|
50.41%
|
n
|
348,800
|
49.59%
|
|
Negativity
|
|
Value
|
n
|
%
|
Value
|
n
|
%
|
Most Polarized
|
-5
|
2,140
|
0.60%
|
-5
|
2,339
|
0.67%
|
|
-4
|
24,431
|
6.89%
|
-4
|
26,154
|
7.50%
|
|
-3
|
34,335
|
9.68%
|
-3
|
42,431
|
12.16%
|
|
-2
|
68,583
|
19.34%
|
-2
|
72,591
|
20.81%
|
Most Neutral
|
-1
|
225,050
|
63.48%
|
-1
|
205,285
|
58.85%
|
|
Positivity
|
|
Value
|
n
|
%
|
Value
|
n
|
%
|
Most Polarized
|
5
|
999
|
0.28%
|
5
|
335
|
0.10%
|
|
4
|
12,002
|
3.39%
|
4
|
5,963
|
1.71%
|
|
3
|
67,187
|
18.95%
|
3
|
43,251
|
12.40%
|
|
2
|
96,583
|
27.24%
|
2
|
98,748
|
28.31%
|
Most Neutral
|
1
|
177,768
|
50.14%
|
1
|
200,503
|
57.48%
|
To develop a cursory understanding of sentiment toward specific topics, we extracted keywords from video titles and descriptions, ignoring stopwords. We then calculated average sentiment for videos that used common keywords and found that some video topics were descriptively more likely to exhibit positive sentiment in comments and replies (e.g., beauty, passion, career), while others appeared much more likely to exhibit negative sentiment (e.g., cancer, college, pain; cf. Fig. 1). Further analysis of these differences exceeded the scope of this study but would likely be fruitful for future research.
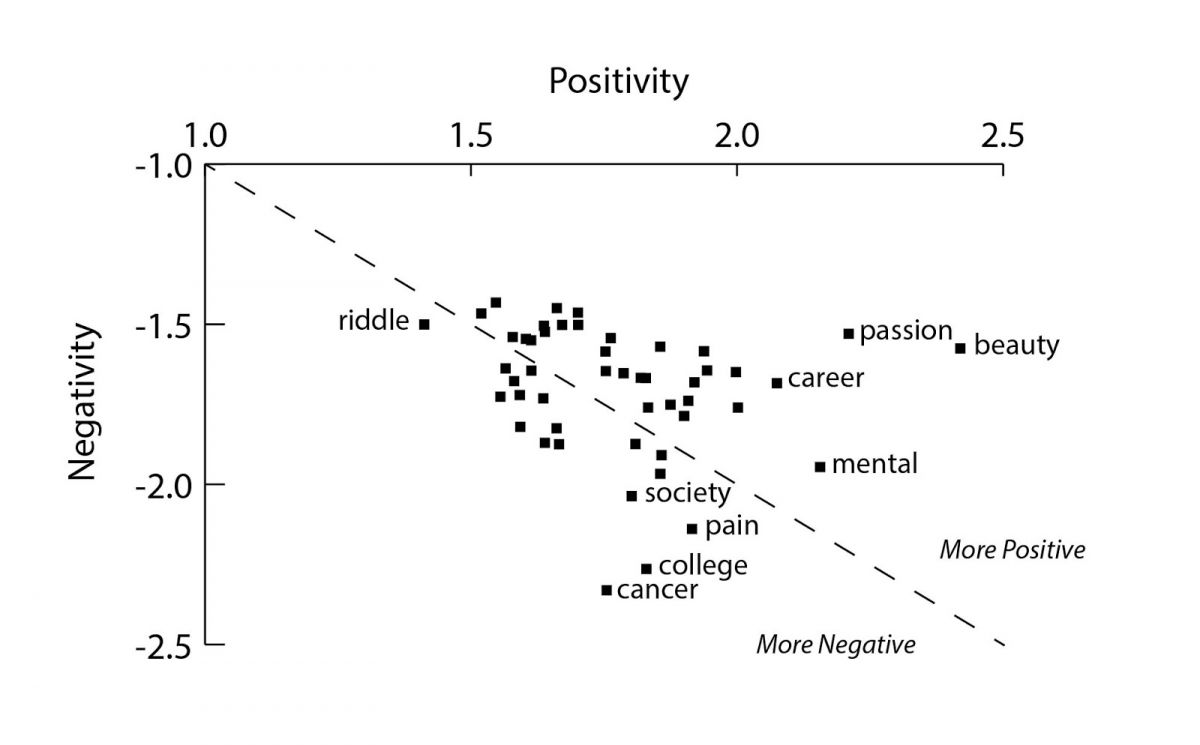
Fig. 1. Polarity and neutrality of some common topical keywords from titles and descriptions
RQ2. Influence of Speaker Gender, Delivery Format, and Comment Threading on Sentiment
Sentiment analysis revealed fairly similar positivity and negativity across comments by gender and delivery format groups, with animations exhibiting the lowest absolute values and female speakers exhibiting the highest (cf. Table 4). Animations also exhibited the lowest variation in values, and female speakers exhibited the highest.
Table 4. Sentiment differences of comments and replies by gender of video presenter
|
Positivity
|
Negativity
|
M
|
SD
|
M
|
SD
|
Animation
|
1.55
|
0.76
|
-1.63
|
0.94
|
Female Speaker
|
1.94
|
0.94
|
-1.83
|
1.09
|
Male Speaker
|
1.82
|
0.90
|
-1.63
|
0.97
|
In addition to these variables, we also anticipated that the sentiment of a reply’s parent comment and the sentiment of previous replies in a comment thread might influence the sentiment of subsequent replies. For example, if one user left a very negative comment on a video, subsequent users replying to that comment might also be very negative as well. Alternatively, subsequent users might post less negative or more positive comments to counteract the original negativity. For this reason, we included the sentiment of each reply’s parent comment and the sentiment of up to four previous replies as lagged variables (e.g., N-1 refers to the reply preceding the studied reply with a lag of 1). Table 5 shows the descriptive statistics of the variables used in the multilevel regression model divided up by level. The reply-level predictors show the statistics for the sentiment level of the previous replies in the thread (up to 5) as well as the cardinality (the number in the thread of the reply). The sample size of the sentiment level of the previous replies decreased as the number of replies increased. This reflects the fact that some of the threads were small and had few replies. This missingness was handled by the FIML technique. The sample size at the different levels reflects the unique number of parent comments and presentation types.
Table 5. Descriptive statistics of variables in multilevel regression model
Variable
|
n
|
Mean
|
Variance
|
Min
|
Max
|
Outcomes
|
Positive Sentiment (PS) of reply
|
348,800
|
1.69
|
0.61
|
1.00
|
5.00
|
Negative Sentiment (NS) of reply
|
348,800
|
-1.70
|
0.98
|
-5.00
|
-1.00
|
Reply Level Predictors
|
PS of previous N-1 reply
|
290,501
|
1.58
|
0.60
|
1.00
|
5.00
|
PS of previous N-2 reply
|
253,731
|
1.58
|
0.60
|
1.00
|
5.00
|
PS of previous N-3 reply
|
226,608
|
1.57
|
0.60
|
1.00
|
5.00
|
PS of previous N-4 reply
|
205,651
|
1.57
|
0.60
|
1.00
|
5.00
|
NS of previous N-1 reply
|
290,501
|
-1.72
|
1.00
|
-5.00
|
-1.00
|
NS of previous N-2 reply
|
253,731
|
-1.73
|
1.01
|
-5.00
|
-1.00
|
NS of previous N-3 reply
|
226,608
|
-1.73
|
1.02
|
-5.00
|
-1.00
|
NS of previous N-4 reply
|
205,651
|
-1.73
|
1.02
|
-5.00
|
-1.00
|
Cardinality of Reply
|
348,800
|
20.19
|
1,684.15
|
1.00
|
500.00
|
Comment Level Predictors
|
Number of replies
|
51,807
|
5.97
|
199.13
|
1.00
|
497.00
|
Parent Comment PS
|
58,107
|
1.70
|
0.73
|
1.00
|
5.00
|
Parent Comment NS
|
58,107
|
-1.84
|
1.12
|
-5.00
|
-1.00
|
Presenter Level Predictors
|
Male Presenter Dummy Variable
|
659
|
0.26
|
0.19
|
0.00
|
1.00
|
Animation Presentation Dummy Variable
|
659
|
0.62
|
0.24
|
0.00
|
1.00
|
The assumptions of linearity, normality, and equality of variance was checked by visual inspection of the histogram of the residuals and the residual plot. This data violated the assumption of normality because of the discrete nature of the data but as the sample size is large, which invokes the central limit theorem, and MPLUS estimates its parameters with the Huber-White correction for non-normality (Huber, 1967) this violation need not be of concern. The assumption of linearity and equality of variance were not violated according to the plots.
Table 2 shows the intraclass correlations (ICCs) and the design effects (DEFFs) of the clustering effects of parent comment and presenter. Muthén and Satorra (1995) demonstrated that a DEFF, which is a function of sample size and the ICCs, is a measure of the effect of clustering on your parameter estimates. If a DEFF is lower than two, then you can ignore that level of clustering. Table 6 shows that the DEFFs for positive and negative sentiment were lower than two at the parent comment level. Nevertheless, this model was still included in the model to more accurately reflect the reality of the situation. The DEFFs for the presenter level were quite high as the average sample size per presenter was also high, meaning that this level needed to be included in the analysis.
Table 6. Design effects of positive sentiment and negative sentiment of replies at the level of parent comment and presenter
|
Intraclass Correlation
|
Average Cluster Size
|
Design Effect
|
Parent Comment Level
|
Positive Sentiment of replies
|
0.06
|
6.28
|
1.33
|
Negative Sentiment of replies
|
0.08
|
6.28
|
1.40
|
Presenter Level
|
Positive Sentiment of replies
|
0.04
|
603.01
|
22.67
|
Negative Sentiment of replies
|
0.06
|
603.01
|
35.31
|
Table 7 shows the multilevel results of the covariates on the positive sentiment and negative sentiment of replies. These results were run simultaneously in an SEM framework allowing positive sentiment and negative sentiment of a reply to covary. The results showed very little predictive power of the covariates at the reply level (R^2=0.01 for positive sentiment, R^2=0.01 for negative sentiment), and the standardized betas for the previous replies’ sentiments were very low. This indicated that the sentiment of previous replies and the cardinality of the reply did not predict the positive or negative sentiment of the subsequent reply.
Table 7. Multilevel Regression results of covariates on positive and negative sentiment of replies
|
Outcome
|
Predictor
|
Positive Sentiment
(higher is more polar)
|
Negative Sentiment
(higher is more neutral)
|
|
B
|
SE
|
β
|
B
|
SE
|
β
|
Reply Level Predictors
|
PS of previous N-1 reply
|
0.05**
|
0.00
|
0.06
|
--
|
--
|
--
|
PS of previous N-2 reply
|
0.05**
|
0.00
|
0.05
|
--
|
--
|
--
|
PS of previous N-3 reply
|
0.02**
|
0.00
|
0.02
|
--
|
--
|
--
|
PS of previous N-4 reply
|
0.02**
|
0.00
|
0.02
|
--
|
--
|
--
|
NS of previous N-1 reply
|
--
|
--
|
--
|
0.08**
|
0.00
|
0.08
|
NS of previous N-2 reply
|
--
|
--
|
--
|
0.06**
|
0.00
|
0.06
|
NS of previous N-3 reply
|
--
|
--
|
--
|
0.02**
|
0.00
|
0.02
|
NS of previous N-4 reply
|
--
|
--
|
--
|
0.02**
|
0.00
|
0.03
|
Cardinality of Reply
|
0.00**
|
0.00
|
0.02
|
-0.00**
|
0.00
|
-0.02
|
R^2
|
0.01**
|
0.00
|
NA
|
0.00**
|
0.00
|
NA
|
Comment Level Predictors
|
Number of replies
|
0.00**
|
0.00
|
-0.03
|
0.00
|
0.00
|
-0.01
|
Parent Comment PS
|
0.07**
|
0.00
|
0.42
|
-0.02**
|
0.00
|
-0.10
|
Parent Comment NS
|
-0.03**
|
0.00
|
-0.24
|
0.11**
|
0.00
|
0.53
|
R^2
|
0.27**
|
0.01
|
NA
|
0.31**
|
0.01
|
NA
|
Presenter Level Predictors
|
Male Presenter Dummy Variable
|
-.07**
|
0.02
|
-0.52
|
0.10**
|
0.03
|
0.62
|
Animation Presentation Dummy Variable
|
-0.21**
|
0.02
|
-1.70
|
0.14**
|
0.02
|
0.87
|
R^2
|
0.44**
|
0.04
|
NA
|
0.08**
|
0.03
|
NA
|
** Indicates significance at the p < .01 level.
The covariates at the parent comment level were more predictive than the reply level (R^2=0.27 for positive sentiment, R^2=0.31 for negative sentiment). The number of replies, did not statistically predict the negative sentiment of a reply (β = -0.01, p = 0.13), and while it did statistically predict positive sentiment, the standardized beta was very small (β = -0.03, p = 0.01). Parent comment positive sentiment was positively predictive of reply positive sentiment (β = 0.42, p < 0.01), meaning that positive comments begat positivity in replies; and parent comment negative sentiment was positively predictive of reply negative sentiment (β = 0.53, p < 0.01), meaning that negative comments begat negativity in replies. Each of these effects accounted for about one-half of a standard deviation in reply sentiment variance. Conversely, parent comment negative sentiment was negatively predictive of reply positive sentiment (β = -0.24, p < 0.00), meaning that less polarity in comment negativity yielded less polarity in reply positivity; and parent comment positive sentiment was weakly negative predictive of reply negative sentiment (β = -0.10, p < 0.01), meaning that polarity in comment positivity also somewhat increased polarity in reply negativity. Taken together, these results indicated that greater polarity in the comment led to greater polarity in replies in all variable instances.
The covariates at the presenter level were predictive of reply positive sentiment (R^2=0.44) but were only weakly predictive of reply negative sentiment (R^2=0.08). Being a male presenter led to greater neutrality in both positivity (β = -0.52, p < 0.01) and negativity (β = 0.62, p < 0.01), meaning that female presenters experienced greater polarity in replies accounting for more than one-half of a standard deviation. Additionally, the animation format neutralized both the negativity (β = 0.87, p < 0.01) and positivity of replies (β = -1.70, p < 0.01) at a very high rate, meaning that the presenter format faces much more positivity and negativity in replies. These results indicated that presenter gender and video format influenced the sentiment of replies all the way down and not just the initial comments that were directed toward the video.
RQ3. Projected Effects of Moderation
To test the effect that increased moderation might have had on the dataset, we compared the number of replies with negative sentiment against others that occurred in response to a negative comment. We found that if moderators had set their offensiveness threshold (i.e., that which they deemed to be too negative for the community) to -5 and deleted comments that met this criteria, it would have prevented only 4.36% of offensive replies and would have inadvertently also prevented 1.46% of all other replies (cf. Table 8). Though this percentage may seem low, it represents preventing non-offensive vs. offensive replies at a rate of 50-to-1, which means that such moderation would have impacted non-offensive replies much more than offensive replies. In contrast, if the offensiveness threshold was set to the very strict level of -2, this would have prevented 62.48% of offensive replies along with 46.63% of non-offensive replies. This represents a much higher occurrence of overall censorship (53.15% of all replies), but it also would reduce the non-offensive to offensive rate at which replies were prevented to almost 1-to-1. Herein the difficulty of comment moderation is made apparent in that the level of moderation must be weighed by its impact on both negative community behaviors (offensive replies) as well as positive community behaviors (non-offensive replies). Notably, however, even by employing the strictest offensiveness threshold available (-2), we were only able to prevent 68.75% of the most offensive replies (-5) and 62.48% of all offensive replies (-2 to -5). For this reason and the fact that replies overall were only slightly more negative than were comments, it seems that comment moderation based on sentiment would not be a reasonable solution to preventing offensive replies in a space like this.
Table 8. Projected effects of comment moderation on preventing offensive replies by threshold (-5 to -2)
Sentiment of Prevented Replies
|
n
|
%
|
Parent Comment Offensiveness Threshold
|
-5
|
-4
|
-3
|
-2
|
-5 (Extremely Negative)
|
2,339
|
0.67%
|
4.36%
|
30.74%
|
48.14%
|
68.75%
|
-4
|
26,154
|
7.50%
|
3.44%
|
32.79%
|
51.05%
|
70.92%
|
-3
|
42,431
|
12.16%
|
2.23%
|
18.68%
|
40.24%
|
63.19%
|
-2
|
72,591
|
20.81%
|
1.51%
|
14.15%
|
30.64%
|
58.83%
|
-1 (Not Negative)
|
205,285
|
58.85%
|
1.07%
|
11.27%
|
24.68%
|
46.63%
|
Total Replies Prevented
|
1.50%
|
14.51%
|
29.95%
|
53.15%
|
Offensive Replies Prevented
|
4.36%
|
32.62%
|
44.49%
|
62.48%
|
Non-Offensive Replies Prevented
|
1.48%
|
12.90%
|
26.24%
|
46.63%
|
Non-Offensive to Offensive Rate
|
50.33 to 1
|
4.45 to 1
|
2.31 to 1
|
1.07 to 1
|
Discussion
In this paper, we examined the sentiment expressed in response to TED talks posted on YouTube, the variables associated with said sentiment, and the projected effects that sentiment-based moderation would have had on posted content. We found that (a) overall most comments and replies are neutral in nature (vis-a-vis positive or negative polarity), (b) some topics are more likely than others to exhibit positive/negative sentiment, (c) videos of male presenters saw greater neutrality in both positivity and negativity, (d) videos of female presenters saw significantly greater polarity in replies, (e) animations neutralized both the negativity and positivity of replies at a very high rate, (f) gender and video format influenced the sentiment of replies and not just the initial comments that were directed toward the video, and (g) using sentiment as a way to moderate offensive content would have a significant effect on non-offensive content. These findings have significant implications.
Results suggest that negativity in comments and replies is common and unpredictable. Though some past scholarship suggests that scholars may potentially face negative experiences online, the bulk of the literature suggests positive outcomes for scholars who choose to participate online. Our research however demonstrates how common and widespread negativity may be in online contexts. Though the results presented here are bounded by the context of the study (i.e. TED talks posted on YouTube), they nonetheless provide a benchmark against which to compare future results in other settings (e.g., negativity on Twitter, Facebook, personal blogs). Furthermore, results suggest that negativity engenders negativity, while positivity engenders positivity (at least somewhat). This finding has significant implications for design and research. Design-wise, one possible approach to reduce negativity and harassment online may be to implement early-warning algorithms that track early signs of negativity to either predict negativity of greater magnitude or to encourage positivity to counteract negative trends. From a research perspective, it would be important to understand not just the reasons why negativity/positivity begets more negativity/positivity, but also the converse: Why do some people “break the mold” and respond positively to a negative thread? What characterizes these individuals? How can we empower more individuals to respond positively to a thread that is characterized by negativity?
Results also suggest that responses to videos featuring female educators will likely exhibit greater polarization and less neutrality than videos of male educators. This finding calls into question the push to encourage all researchers to be active on social media (citation). As expected, gender mediates reactions to online participation. We urge those who encourage and prepare faculty to participate online (e.g., faculty developers and social media trainers) to recognize that male and female faculty will have different experiences online and to help faculty recognize that their online participation may have differential effects based on their gender. Further research into this finding is necessary. What other variables influence polarization? To what degree, for example, does the topic examined by the presenter influence polarization? Would a female speaker face greater polarization than a male speaker when discussing certain topics (e.g., religion or feminism)? It is also significant to note here that more in-depth qualitative analysis of the results presented here may shed more light into the sentiment expressed toward YouTube videos. For instance, while we might be tempted to view increased positivity in a desirable light, it is possible that upon closer inspection we realize that increased positivity might reflect a form of harassment (e.g., in the form of cat-calling or similar behaviors).
One strategy that individuals who want a more neutral experience could employ may be to utilize an alternative video format. Results suggest that videos featuring animations as opposed to male/female speakers exhibited more neutral comments, with 0.8 S.D. less negativity and 1.7 S.D. less positivity. Therefore, in practical terms, if speakers anticipate a strong negative reaction to their work, one way to lessen such anticipated reaction might be to present their findings online in animated form. Such practice, however, puts the onus on the presenter, and we believe that this is an issue that requires the attention of comment/reply contributors much more than the attention of presenters. Indeed, to reduce the incidence of negativity online it would seem prudent to expand our conversations around civility, compassion, empathy, respectful dialogue, and acceptance to include everyone who participates online and not simply advise content creators to change the ways they behave, speak, or present their content. Such conversations may be had at home, but also in classrooms, schools, and religious gatherings.
Finally, these results reveal that moderating comment threads has a complicated effect on participation and that such moderation does not necessarily prevent future negativity. Overly strict moderation is likely to lead to much more collateral damage and reduce positivity. Thus, algorithms and technologies developed to automate moderation and combat offensive content should extend beyond sentiment analysis. We do wonder however whether the efforts to moderate and limit negativity reflects a larger trend of allowing negative outliers in these huge datasets of online participation to influence content creators more than the masses. Like qualitative researchers, content creators may be drawn to the outliers (e.g., trolls) as informants, and this might shape their perceptions disproportionately to the typical user. Though extremely negative commenters represent a minority of participants identified in the dataset collected here, if their effect on content creators is significant, they may end up controlling what happens online. It is significant to note that not all comments are created equal or have an equal influence on our perception and reactions, and negative comments (e.g., comments that remind the author of previous trauma, such as sexual violence) may have a substantial and lasting impact.
This research opens a number of significant future research directions for researchers vested in understanding and improving online interactions. Significantly, this is a topic of interest to a wide array of disciplines and researchers from education, sociology, media and communication studies, human-computer interaction, and computer science may contribute significantly to a multidisciplinary understanding of the phenomenon. For example, it seems that researchers need to develop a greater understanding of the relationship between sentiment and civility. Is sentiment in language a predictor or an outcome of civil online behavior? Looking beyond sentiment, how do offensive, threatening, and profane comments impact participation, future replies, and presenters? Are there observable differences in how language is employed to describe women vs. men in comments to online videos? Do we see similar outcomes in other settings? What topics are most likely to generate polarity in online discussions? How should scholars and educators prepare doctoral students, early-career researchers, and academics new to public online spaces to navigate this space effectively and face negative sentiment online? What strategies can scholars use to curtail online negativity directed at them? What are the ethical responsibilities for training academics to use social media and the public web for scholarship when emerging research suggests that some research areas are rife with tensions and are likely to encounter unsavory audiences online? This research provides a starting point for delving deeply into the significant effects discovered here, and the questions posed provide fertile ground for future research.
References
Berrios, R., Totterdell, P., & Kellett, S. (2015). Eliciting mixed emotions: a meta-analysis comparing models, types, and measures. Frontiers in Psychology, 6, 428. https://edtechbooks.org/-gVms
Bjorklund, W. L., & Rehling, D. L. (2009). Student perceptions of classroom incivility. College Teaching, 58, 15–18. http://doi.org/10.1080/87567550903252801
Bollen, J., Mao, H., & Pepe, A. (2011). Modeling public mood and emotion: Twitter sentiment and socio-economic phenomena. In Fifth International AAAI Conference on Weblogs and Social Media (pp. 450–453). Barcelona.
Cheng, J., Bernstein, M., Danescu-Niculescu-Mizil, C., & Leskovec, J. (2017). Anyone can become a troll: Causes of trolling behavior in online discussions. In Proceedings of the ACM Conference on Computer Supported Cooperative Work and Social Computing. Portland, OR. http://doi.org/10.1145/2998181.2998213
Conole, G., & Alevizou, P. (2010). A literature review of the use of Web 2.0 tools in Higher Education. A report commissioned by the Higher Education Academy. Retrieved from https://www.heacademy.ac.uk/system/files/conole_alevizou_2010.pdf
DaVia Rubenstein, L. (2012). Using TED talks to inspire thoughtful practice. The teacher educator, 47(4), 261-267.
Dodds, P. S., & Danforth, C. M. (2010). Measuring the happiness of large-scale written expression: Songs, blogs, and presidents. Journal of Happiness Studies, 11(4), 441–456. http://doi.org/10.1007/s10902-009-9150-9
Duggan, M. (2014, October). Online harassment. Pew Research Center. Retrieved from http://www.pewinternet.org/2014/10/22/online-harassment/
Flaxman, S., Goel, S., & Rao, J. M. (2013). Ideological segregation and the effects of social media on news consumption. ” SSRN Scholarly Paper ID 2363701, Social Science Research Network. Retreived from https://bfi.uchicago.edu/sites/default/files/research/flaxman_goel_rao_onlinenews.pdf
Flaxman, S., Goel, S., & Rao, J. (2016). Filter bubbles, echo chambers, and online news consumption. Public Opinion Quarterly, 80, 298-320.
Goodyear, P. (2005). Educational design and networked learning: Patterns, pattern languages and design practice. Australasian Journal of Educational Technology, 21(1), 82-101.
Greenhow, C., Robelia, B., & Hughes, J. E. (2009). Learning, teaching, and scholarship in a digital age Web 2.0 and classroom research: What path should we take now?. Educational researcher, 38(4), 246-259.
Han, S., & Brazeal, L. M. (2015). Playing nice: Modeling civility in online political discussions. Communication Research Reports, 32(1), 20–28. http://doi.org/10.1080/08824096.2014.989971
Harlow, S. (2015). Story-chatterers stirring up hate: Racist discourse in reader comments on U.S. newspaper websites. Howard Journal of Communications, 26(1), 21–42. http://doi.org/10.1080/10646175.2014.984795
Huang, K. T., Cotten, S. R., & Rikard, R. V. (2017). Access is not enough: the impact of emotional costs and self-efficacy on the changes in African-American students’ ICT use patterns. Information, Communication & Society, 20(4), 637-650.
Huber, P. J. (1967, June). The behavior of maximum likelihood estimates under nonstandard conditions. In Proceedings of the fifth Berkeley symposium on mathematical statistics and probability (Vol. 1, No. 1, pp. 221-233).
Hughey, M. W., & Daniels, J. (2013). Racist comments at online news sites: a methodological dilemma for discourse analysis. Media, Culture & Society, 35(3), 332–347. http://doi.org/10.1177/0163443712472089
Jenkins, H. (2006a). Convergence culture: Where old and new media collide. New York, NY: NYU press.
Jenkins, H. (2006b). Fans, bloggers, and gamers: Exploring participatory culture. New York, NY: NYU Press.
Kramer, A. D. I. (2010). An unobtrusive behavioral model of “gross national happiness.” Proceedings of the SIGCHI Conference on Human Factors in Computing Systems, 287–290. http://doi.org/10.1145/1753326.1753369
Ksiazek, T. B., Peer, L., & Lessard, K. (2016). User engagement with online news: Conceptualizing interactivity and exploring the relationship between online news videos and user comments. New Media & Society, 18(3), 502–520. https://edtechbooks.org/-ffg
Kwon, K. H., & Gruzd, A. (2017). Is aggression contagious online? A case of swearing on Donald Trump’s campaign videos on YouTube. In Proceedings of the 50th Hawaii International Conference on System Sciences. Retrieved from http://scholarspace.manoa.hawaii.edu/handle/10125/41417
Lenhart, A., Ybarra, M., Zickuhr, K., & Price-Feeney, M. (2016). Online harassment, digital abuse, and cyberstalking in America. New York: Data & Society Research Institute.
Little, R. J., & Rubin, D. B. (2014). Statistical analysis with missing data. John Wiley & Sons.
Luke, D. A. (2004). Multilevel modeling (Vol. 143). Sage.
Lowenthal, P. R., Dunlap, J. C., & Stitson, P. (2016). Creating an intentional web presence: Strategies for every educational technology professional. TechTrends, 60(4), 320-329.
Muddiman, A., & Stroud, N. J. (2017). News values, cognitive biases, and partisan incivility in comment sections. Journal of Communication, 1–24. http://doi.org/10.1111/jcom.12312
Muthén, L.K. and Muthén, B.O. (1998-2015). Mplus User’s Guide. Seventh Edition. Los Angeles, CA: Muthén & Muthén
Muthén, B. O., & Satorra, A. (1995). Complex sample data in structural equation modeling. Sociological methodology, 25, 267-316.
Oksanen, A., Garcia, D., Sirola, A., Näsi, M., Kaakinen, M., Keipi, T., & Räsänen, P. (2015). Pro-anorexia and anti-pro-anorexia videos on YouTube: Sentiment analysis of user responses. Journal of medical Internet research, 17(11).
Pang, B., & Lee, L. (2008). Opinion mining and sentiment analysis. Foundations and Trends in Information Retrieval, 2(1–2), 1–135.
Pang, B., Lee, L., & Vaithyanathan, S. (2002). Thumbs up?: Sentiment classification using machine learning techniques. In Proceedings of the Conference on Empirical Methods in Natural Language Processing (pp. 79–86). Stroudsburg, PA. http://doi.org/10.3115/1118693.1118704
Reader, B. (2012). Free press vs. free speech? The rhetoric of “Civility” in regard to anonymous online comments. Journalism & Mass Communication Quarterly, 89(3), 495–513. http://doi.org/10.1177/1077699012447923
Robinson, L., Cotten, S. R., Ono, H., Quan-Haase, A., Mesch, G., Chen, W., ... & Stern, M. J. (2015). Digital inequalities and why they matter. Information, Communication & Society, 18(5), 569-582.
Romanelli, F., Cain, J., & McNamara, P. J. (2014). Should TED talks be teaching us something?. American journal of pharmaceutical education, 78(6), 113.
Shirky, C. (2008). Here comes everybody: The power of organizing without organizations. New York, NY: Penguin.
Steeples, C., Jones, C., & Goodyear, P. (2002). Beyond e-learning: A future for networked learning. In C. Steeples & C. Jones (Eds.), Networked learning: Perspectives and issues (pp. 323-341). London: Springer.
Tess, P. A. (2013). The role of social media in higher education classes (real and virtual)–A literature review. Computers in Human Behavior, 29(5), A60-A68.
Thelwall, M., Buckley, K., & Paltoglou, G. (2011). Sentiment in Twitter events. Journal of the American Society for Information Science and Technology, 62(2), 406–418. https://edtechbooks.org/-xWS
Tsou, A., Thelwall, M., Mongeon, P., & Sugimoto, C. R. (2014). A community of curious souls: an analysis of commenting behavior on TED talks videos. PloS one, 9(4), e93609.
Turney, P. D. (2002). Thumbs up or thumbs down? Semantic orientation applied to unsupervised classification of reviews. In Proceedings of the 40th Annual Meeting of the Association for Computational Linguistics (ACL) (pp. 417–424). Philadelphia, PA. http://doi.org/10.3115/1073083.1073153
Van Dijk, J. (2012). The network society (3rd ed.). Thousand Oaks, CA: Sage.
Veletsianos, G., & Kimmons, R. (2012). Networked participatory scholarship: Emergent techno-cultural pressures toward open and digital scholarship in online networks. Computers & Education, 58(2), 766-774.
Yates, S., Kirby, J., & Lockley, E. (2015). Digital media use: differences and inequalities in relation to class and age. Sociological research online, 20(4), 12.
Walther, J. B., Deandrea, D., Kim, J., & Anthony, J. C. (2010). The influence of online comments on perceptions of antimarijuana public service announcements on YouTube. Human Communication Research, 36(4), 469–492. http://doi.org/10.1111/j.1468-2958.2010.01384.x
Wang, J., & Wang, X. (2012). Structural equation modeling: Applications using Mplus. John Wiley & Sons.
Waters, A. (2016). Claim your domain--And your online presence. Bloomington, IN: Solution Press.
Weller, M. (2011). The digital scholar: How technology is transforming scholarly practice. New York, NY: Bloomsbury.
Winkelman, S. B., Early, J. O., Walker, A. D., Chu, L., & Yick-Flanagan, A. (2015). Exploring Cyber Harassment among Women Who Use Social Media. Universal Journal of Public Health, 3(5), 194.
Ybarra, M. L., & Mitchell, K. J. (2008). How risky are social networking sites? A comparison of places online where youth sexual solicitation and harassment occurs. Pediatrics, 121(2), e350-e357.