A Theoretical Framework for Defining, Implementing, and Evaluating Personalized Learning
For over a decade, the United States National Educational Technology Plan has called for an increase in personalized learning across P–12 contexts in response to the increasing diversity of learners’ backgrounds, abilities, needs, and interests. Following emergency remote teaching due to the COVID-19 pandemic, the diversity of learners’ abilities and needs may become even more extreme as many learners were ill-prepared for the self-regulation and increased agency of distance learning. As learners become increasingly diverse, it seems clear that a “one-size-fits-all” approach to learning will not work effectively. Based on the need for personalized learning across P–12 contexts, interviews about personalization practices with 62 P–12 teachers, and in response to broad perceptions of personalization across educational fields, the Personalized Learning Design Framework was created to describe elements of instruction that can be personalized, dimensions along which such elements can be personalized, the role technology may play in personalization, and a taxonomy of learner agency to guide a transition from instructor-centered to learner-centered practices.
Personalized learning (PL) has gained nearly unprecedented attention as an educational practice. The United States Department of Education’s (2010, 2017) National Educational Technology Plan and non-profit organizations such as the Bill and Melinda Gates Foundation (2014) and Chan Zuckerberg Initiative (Boninger et al., 2019) have called for increased attention toward preparing P–12 teachers to personalize instruction. Generally speaking, PL involves tailoring instruction to meet individual learners’ needs, abilities, and/or interests. However, the definition of PL has often been broad and ambiguous, making implementation difficult. As expressed by Fisher (2019), “personalized learning” has been used to refer to a “host of efforts and models” (para. 4). The U.S. Department of Education (2010) described PL as any adaptation of instruction based on learners’ needs, interests, or abilities. Other resources have additionally described it as instruction that is tailored to ensure mastery (Patrick et al., 2013); enables students to control instructional choices (Bill & Melinda Gates Foundation et al., 2014; National Center for Learning Disabilities, 2017; LEAP Innovations, 2021), creates strong connections between learners and others (National Center for Learning Disabilities, 2017), adapts the pace of learning (U.S. Department of Education, 2017), makes learning more relevant or individually meaningful (LEAP Learning Framework, 2021; U.S. Department of Education, 2017), engages learners (Knowledge Works, 2022), and/or presents learning plans for each individual learner (Morin, 2021). When definitions of an instructional practice vary so widely, implementation and design of that practice becomes difficult. A design-oriented definition of PL is needed to guide the implementation of instruction that is effectively tailored to learners’ needs, abilities, and/or interests.
There are several reasons tailoring instruction is important to educational contexts. Wells (2020) noted that more than 50% of school-age children in the United States are now students of color, but relatively little has been done to address the increasing cultural diversity in P–12 classrooms – a diversity that students will carry into higher education and the workforce. In addition to increased cultural diversity, educators also face increasing academic diversity. While some academic diversity may be expected, the ongoing problem regarding the existence of achievement gaps has been well documented (see Dover, 2009). We can expect even wider achievement gaps when learners face large-scale disruptions such as the COVID-19 pandemic (Pokhrel & Chhetri, 2021). A 2022 School Pulse Panel study from the National Center for Education Statistics found that 89% of public-school staff and 82% of public-school parents were concerned about learners’ ability to meet academic standards during the 2021–2022 school year.
As the field of education adjusts to increased cultural and academic diversity, educators will need to understand how to provide instruction tailored to learners’ diverse backgrounds, abilities, needs, and interests to provide targeted and meaningful learning interventions. Additionally, PL needs to provide a scaffold for increasing learner agency to develop learners who are better prepared to increase their ownership over their learning. Increased learner ownership has the potential to promote learning during emergency learning situations, self-directed learning, and/or life-long learning. A framework for designing PL is essential to twenty-first century learning because educators will need to create instructional activities and use instructional modalities that break away from one-size-fits-all and teacher-centered approaches to learning.
A Vision for Personalized Learning
A clear vision is needed to determine how instruction can be personalized, what personalization is based on, and who or what personalizes instruction. Shemshack and Spector (2020) explained that “personalized learning cannot be a solution to learning until it is defined better and developed more thoroughly,” adding that “personalized learning for everyone looks different according to the needs and goals of the individual” (p. 17). Educators should not be surprised by the multitude of approaches to describing PL. Gibbons (2013) suggested that “theorists who have strategy concepts to promote often use the [strategy’s] term[inology] opportunistically to refer to their particular interests without cross-referencing their ideas with those of others” (p. 33). The Personalized Learning Design Framework (PLDF) presents a framework that is based on the cross-refencing of approaches to PL and provides descriptions of what Graham et al. (2013) referred to as the “core attributes” of the pedagogical layer of instruction. As such, the framework accounts for the relationship between personalized learning and learner agency to scaffold learners to appropriate ownership over their learning. The PLDF provides a vision for defining, designing, and evaluating PL by addressing the following questions:
- What aspect of instruction is being tailored to the learner – learning objectives, assessments of learning, or learning activities?
- Along which dimensions of PL is instruction being tailored to the learner – time, place, pace, path, and/or goals of learning?
- Who or what is tailoring the instruction – the educator, the learner, or an instructional application/system?
- At what level of the Taxonomy of Learner Agency is instruction being tailored to the learner – is the instructor differentiating instruction (Level 2), providing learning options for the learner to select from (Level 3), or guiding learners in creating their own learning options (Level 4)?
- What kind of data is used to inform the tailoring of instruction – performance, activity, and/or learner profile data?
Instructional Elements and Dimensions of Personalized Learning
Short and Graham (in review) interviewed 62 P–12 teachers about their personalized learning implementation. These interviews provided rich data about the transforming potential of blended learning (Graham et al., 2022; Hanny et al., 2021). Regarding PL, teachers were asked to describe how their learners were given voice and choice over their learning. Short and Graham (in review) uncovered that, regardless of context, teachers offered personalization of instruction across three elements of instruction (see Figure 1) and five dimensions of personalization (see Figure 2). These elements of instruction and dimensions of PL provide two of the core attributes of PL.
Figure 1
Personalized Learning Instructional Elements
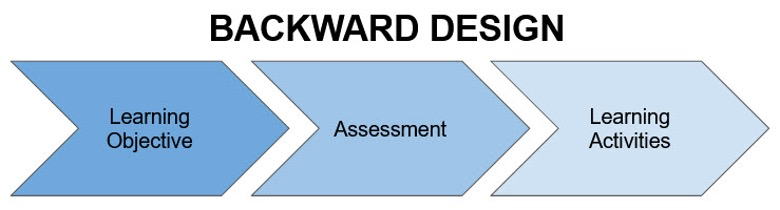
All 62 teachers interviewed by Short and Graham (in review) allowed for personalization of learning activities, 51 for personalization of assessments, and 24 for personalization of learning objectives. Learning objectives were mostly personalized within contexts that don’t have state-mandated learning objectives (e.g., technology, arts, and support staff), but at least one teacher within each context offered personalization of learning objectives. Multiple teachers from each context personalized assessments and activities. The researchers found that these elements were personalized along the PL dimensions presented by Graham, Borup, Short, and Archambault (2019).
Graham, Borup, Short, and Archambault (2019) described personalized learning as one of the four research-based competency areas of blended teaching (Archibald et al., 2021; Graham et al., 2017; Graham et al., 2018; Graham, Borup, Pulham, & Larsen, 2019; Pulham et al., 2018; Pulham & Graham, 2018). They described PL based on the definition of blended learning from Horn and Staker (2017), which describes a pedagogy that provides students with some control over the time, place, pace, and/or path of learning. Graham, Borup, Short, and Archambault (2019) added a fifth dimension to personalized learning: goals. Practical implications for PL across instructional elements and dimensions of PL are provided in the implementation section of this paper.
Figure 2
Dimensions of Personalized Learning from Graham, Borup, Short, and Archambault (2019)

Who or What Provides Tailored Instruction
While some descriptions of PL require that instruction be learner-driven, this requirement is not ubiquitous. Even definitions that agree PL should be learner-driven, disagree on how much of it should be learner-driven. For example, the National Educational Technology Plan (U.S. Department of Education, 2017) states that PL is “often self-initiated” (p.9), while the LEAP Learning Framework requires that PL be “led with and demonstrated by the learner” (2021, para. 2). Because PL is perceived to be either teacher-driven or learner-driven, the PLDF establishes a description of who or what is tailoring instruction. Instruction can be tailored by educators, learners, or adaptive learning applications (see Figure 3). An adaptive learning application uses performance data to determine whether a learner needs to review previous materials or if a learner is ready to advance. The application then provides learners with instruction it deems appropriate.
Figure 3
Possible sources of tailored instruction – the educator, the learner, and technology.
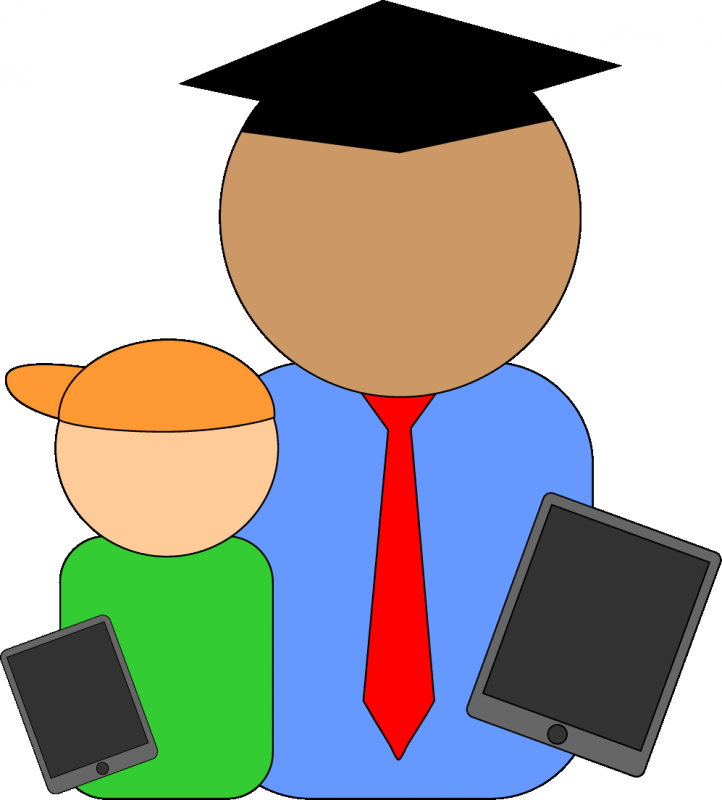
It is important for educators to consider the role of the learner in tailoring instruction. Many descriptions of PL include an increase in learner agency; however, in many cases, it may be irresponsible to ask some learners to make learning decisions without helping them develop the skills required for doing so. I developed the Taxonomy of Learner Agency (see Figure 4) to help learners navigate the choices that come with increased agency over their learning.
Figure 4
The Taxonomy of Learner Agency
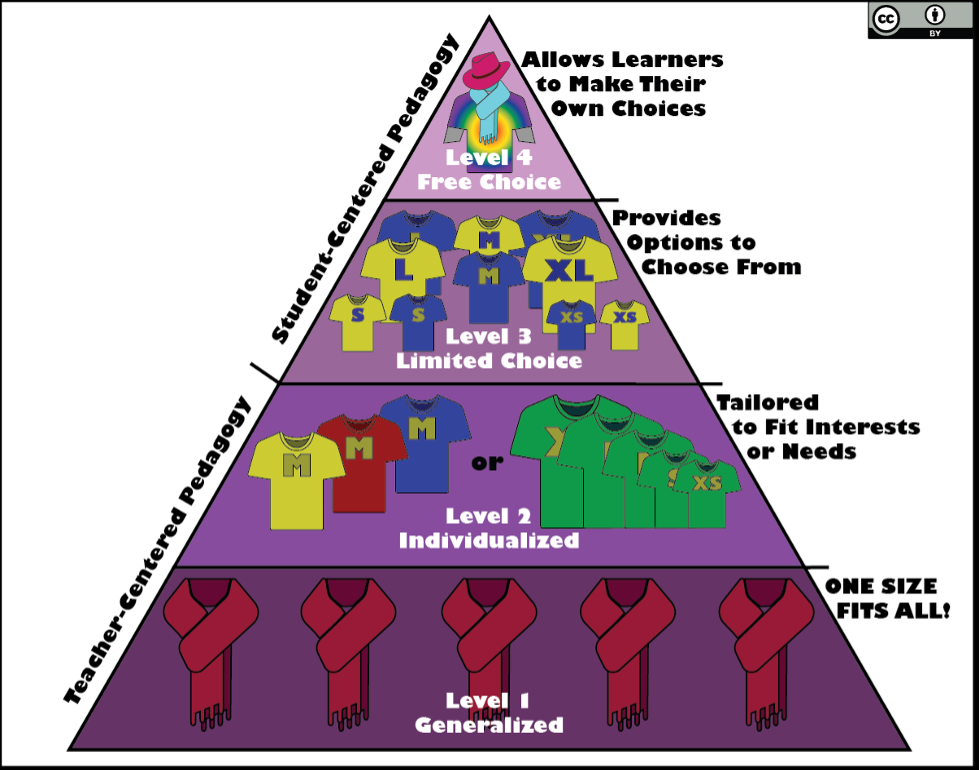
The Taxonomy of Learner Agency provides four levels for scaffolding and transitioning from teacher-centered pedagogy that presents a one-size-fits-all approach to a personalized approach where the learner makes informed decisions regarding the time, place, pace, path, and/or goals of their learning objectives, assessments, or learning activities. The Taxonomy of Learner Agency can be compared to Moore’s (1993) transactional distance theory which provided degrees of autonomous learning along the elements of goals, evaluation, and execution – what the PLDF identifies as learning outcomes, assessment, and learning activities. The PLDF builds on Moore’s (1993) theory by identifying the dimensions (time, place, pace, path, and/or goals) in which autonomy can be created within instructional elements. Table 1 provides a description of each level of the Taxonomy of Learner Agency.
Table 1
Levels of Personalization Autonomy
Level of Personalization |
Description |
Level 1 |
No adaptation of instruction – instruction is uniform for all students. |
Level 2 |
Instruction is individualized or differentiated – the instructor tailors instruction for some students based on students’ needs, abilities, or interests. |
Level 3 |
Students have some choice – they are given some control over their learning by selecting from options or working within parameters across learning goals, time, place, pace, and/or path. |
Level 4 |
Students have guided autonomy – students create their own goals, time, place, pace, and/or path of learning with minimal instructor guidance or oversight. |
Using Data to Create Informed Personalized Learning
Another important aspect of defining, designing, and evaluating PL is creating data-driven instruction. Instruction can be tailored in many ways for many reasons. Educators should use performance data, activity data, and learner profile data to validate and inform their PL opportunities. I collectively refer to these types of data as PAL data (see Figure 5). Performance data consists of knowledge or ability measurements. Activity data represents a learner’s learning behaviors and habits. Learner profile data is usually qualitative data used to understand a learner’s interests and background. Educators need to know which kind of PAL data is the most appropriate to answer various instructional questions. They also need to be equipped with the ability to effectively analyze PAL data, looking for patterns that inform PL opportunities.
Figure 5
A representation of the data types that can be used to tailor instruction.
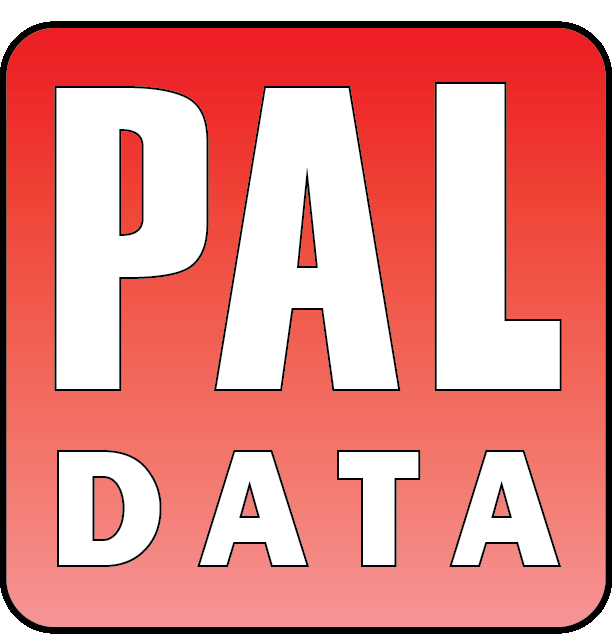
Implementation of the Personalized Learning Design Framework
The PLDF brings together instructional elements of PL with the dimensions of PL to create data-driven instruction that can address individual learners’ needs, abilities, and interests while promoting learner agency. PL is not a new approach to instruction, but technology provides educators with the resources needed to implement it more easily. Examples of the PLDF in practice are provided below.
Personalized Learning in Practice
A clearer vision of what constitutes PL is a necessary foundation for designing and implementing PL; however, examples of PL in practice can further solidify this foundation. The following sections build on the descriptions of PL above by providing real-world examples of PL in various contexts, separated by various implementations of PL. The first section describes the intersection between tailoring instructional elements and dimensions of personalization to learners’ backgrounds, abilities, needs, and interests. The second section provides examples of using PAL data in PL. The last section describes PL at various levels of learner agency.
Personalizing Instructional Elements and Dimensions in Practice
Educators can tailor the time, place, pace, path, and/or goals of learning objectives, assessments, and/or learning activities. Table 2 presents some practical examples of PL across instructional elements and dimensions of PL. An important distinction between goals and learning objectives is that learning objectives almost always focus on demonstrating knowledge or ability, while goals often focus more on learning habits and behaviors. The personalization of goals may also direct the other four dimensions of PL.
Table 2
Personalization of Instructional Elements Across Personalization Dimensions
Personalization Dimension |
Instructional Elements |
Learning Objective |
Assessment |
Instructional Activities |
Goals |
Learners determine strategies for setting objectives, or design parameters to guide other personalization dimensions. |
Learners set achievement goals (e.g., earning an 80%), make a plan for implementing testing strategies, or design parameters to guide other personalization dimensions. |
Learners choose how they will stay on task while completing activities, or design parameters to guide other personalization dimensions. |
Time |
Learners choose when an objective will be met or started. |
Learners choose when to demonstrate learning. |
Learners choose when to complete activities. |
Place |
Learners determine where or with whom learning will occur. |
Learners choose where or with whom to demonstrate learning. |
Learners choose where or with whom to complete activities. |
Pace |
Learners choose how quickly learning objectives will be met. |
Learners choose how quickly to complete a demonstration of learning. This can include multiple attempts. |
Learners choose how quickly to complete activities. |
Path |
Learners choose between multiple learning objectives or the order of completing learning objectives. |
Learners choose how to demonstrate learning, such as what tools to use or the methods of demonstration. |
Learners choose between different learning activities and resources, or create their own. |
PAL Data in Practice
Educators have long used performance data to inform instruction. However, the introduction of adaptive learning software has made data literacy necessary for twenty-first century teaching. Adaptive learning software is a great instructional resource, but educators must understand how to use performance data from software to connect digital learning and in-person learning. Performance data alone, however, is seldomly enough to determine a learner’s background, needs, abilities, and interests.
Activity data can be gathered through educators’ observations of a learner’s time on task, distractions, or preferences. Activity data can also be gathered from electronic sources. Most learning management systems provide data concerning a learner’s time on activities, attempts on assessments, missing assignments, etc. Activity data can indicate that something other than a lack of understanding or ability affected a learner’s performance, such as rushing through an assessment or being absent during a particular lesson.
Learner profile data can be gathered using learner profile surveys and various learner-educator interactions. Educators may use a learner profile survey at the beginning of a semester to get a sense of a learner’s hobbies, interests, friends, aspirations, background, or preferred learning activities and environment. More frequent learner profile surveys can track learners’ social-emotional status, physical needs (shelter, food, etc.), or goals for an instructional period. Using a learner profile as a goal-setting activity can allow learners to state what they want to accomplish during an instructional activity. Learners can reflect on their goal and what helped or harmed their learning progress. Such a reflection can be an important step in increasing learner ownership and agency.
Learner Agency in Personalized Learning
The Taxonomy of Learner Agency provides a focus on the learner’s role in PL. If schools want to create life-long learners, they need to provide learning opportunities that are learner-driven. However, asking learners to make instructional decisions without providing adequate support can be detrimental to their academic progress (see Brockett, 2006; Iyengar & Lepper, 2000; Schwartz, 2004; Schwartz & Ward, 2004; Waterschoot et al., 2019). Yet, with appropriate levels of support and a gradual increase in agency across the dimensions described above, learners can become more motivated and self-aware, and display higher levels of achievement (see Assor, 2012; Bergdahl & Bond, 2021; Fraumeni-McBride, 2017; Schneider et al., 2022). Implementation examples for the Taxonomy of Learner Agency are described below.
- Level 1 - Generalized Instruction. There are times when whole-group instruction can be important, even during PL. As learners gain more agency, instruction at Level 1 may become more managerial than informational. Instruction at this level could include group lectures or presentations, or modeling of correct procedures.
- Level 2 - Individualized Instruction. Using IEP or 504 plans, creating personalized playlists, or using adaptive software provides learners with an understanding that they have individual needs, abilities, and interests that can direct learning. Some examples of instruction at this level include assigning specific training modules to different learners who need to improve upon their unique weaknesses or tailoring instruction based on physical, social, or psychological needs.
- Level 3 - Limited Choice. Providing students with options, such as various levels of mastery to work toward, various forms of assessment, or various videos to watch as part of their learning activities, allows learners to practice their agency in appropriate ways. This lays the groundwork for the learning they will do when an educator may not be in front of them to provide instruction. Instruction at this level includes providing learners with a list of modules or resources to choose from in order to prepare for an upcoming assessment, allowing learners to choose how they can demonstrate their learning, etc.
- Level 4 - Free (but guided) Choice. It may be uncommon in P–12 contexts for students to reach this level all the time, but there are many opportunities for P–12 learners to practice this level of agency. For example, students may freely choose the topic of an essay, whom to partner with for a project, or the format and function of a project as part of a senior project or independent learning time. Some learners may need more guidance at this level than others. It may also be appropriate to take some learners back to Level 3 of the taxonomy if they face choice paralysis. In higher education and corporate settings, instruction at this level may include allowing learners to set their own goals within a period of instruction or development, and then supporting them in meeting those goals by providing adequate and appropriate instructional resources.
Implications for Teaching, Training, and Teacher Education
Instructors and designers often want to create instruction that encourages learning beyond the bounds of instruction. P–12 teachers seek to inspire life-long learning. College instructors seek to nourish an academic curiosity. Human resources and professional development trainers seek to promote on-the-job learning beyond initial training. If designers of such instruction want learners to develop learner-driven habits, it would follow that learners need experience with autonomous, self-directed learning. The PLDF provides a theoretical foundation for creating and scaffolding instruction that nourishes student autonomy within various instructional elements across various dimensions of personalization. According to this framework, the following questions must be addressed when creating PL.
- What aspect of instruction is being tailored to the learner –learning objectives, assessments of learning, or learning activities?
- Along which dimensions of PL is instruction being tailored to the learner – time, place, pace, path, and/or goals of learning?
- Who or what is tailoring the instruction – the educator, the learner, or an instructional application/system?
- At what level of the Taxonomy of Learner Agency is instruction being tailored to the learner – is the instructor differentiating instruction (Level 2), providing learning options for the learner to select from (Level 3), or guiding learners in creating their own learning options (Level 4)?
- What kind of data is used to inform the tailoring of instruction – performance, activity, and/or learner profile data?
Learners are growing more diverse. Twenty-first century educators need to be equipped to provide instruction that can meet diverse backgrounds, abilities, needs, and interests. Educators will need to have the knowledge and ability to develop data-driven instruction that allows learners to experience ownership over their learning. The PLDF creates a shared understanding of PL in practice and provides a foundation for creating and scaffolding instruction that nourishes learner autonomy within various instructional elements, across various dimensions of personalization, and based on various data sources. Future work in this area should focus on using the PLDF to implement PL in various educational contexts, identifying the core attributes of the PLDF that have the greatest impact on learning and development, variation of the PLDF across educational contexts, and creating evaluation matrices that can better guide the development and description of PL practices based on the core attributes of the PLDF.
References
Archibald, D. E., Graham, C. R., & Larsen, R. (2021). Validating a blended teaching readiness instrument for primary/secondary preservice teachers. British Journal of Educational Technology, 52(2), 536-551.
Assor, A. (2012). Allowing choice and nurturing an inner compass: Educational practices supporting students’ need for autonomy. In Handbook of research on student engagement (421-439). Springer.
Bergdahl, N. & Bond, M. (2022). Negotiating (dis-)engagement in K–12 blended learning. Education and Information Technologies, 27(2), 2635–2660. https://doi.org/10.1007/s10639-021-10714-w
Bill & Melinda Gates Foundation, Afton Partners, Eli & Edythe Broad Foundation, CEE Trust, Christensen Institute, Charter School Growth Fund, EDUCAUSE, iNACOL, The Learning Accelerator, Michael & Susan Dell Foundation, & Silicon Schools. (2014). A working definition of personalized learning. Accessed on September 23, 2022. https://assets.documentcloud.org/documents/1311874/personalized-learning-working-definition-fall2014.pdf
Brockett, R. G. (2006). Self-directed learning and the paradox of choice. International Journal of Self-Directed Learning, 3(2), 27–33.
Boninger, F., Molnar, A., & Saldaña, C. M. (2019). Personalized learning and the digital privatization of curriculum and teaching. National Educational Policy Center. https://nepc.colorado.edu/publication/personalized-learning
Dover, A. G. (2009). Teaching for social justice and K–12 student outcomes: A conceptual framework and research review. Equity & Excellence in Education, 42(4), 506–524. https://doi.org/10.1080/10665680903196339
Fisher, J. F. (2019, Jan. 15). Defining personalized learning and blended learning: Is there a difference? Clayton Christensen Institute. https://www.christenseninstitute.org/blog/defining-personalized-learning-and-blended-learning-is-there-a-difference/
Fraumeni-McBride, J. P. (2017). The effects of choice on reading engagement and comprehension for second- and third-grade students: An action research report. Journal of Montessori Research, 3(2), 19-38.
Gibbons, A. S. (2013). An architectural approach to instructional design. Routledge.
Graham, C. R., Borup, J., Jensen, M.., Arnesen, K. T., & Short, C. R. (2022). K-12 Blended Teaching (Vol 2): A Guide to Practice Within the Disciplines. EdTech Books. https://edtechbooks.org/k12blended2
Graham, C. R., Borup, J., Pulham, E., & Larsen, R. (2017). K–12 blended teaching readiness: Phase 1 - instrument development. Lansing, MI. https://mvlri.org/wp-content/uploads/2017/11/k12-blended-teaching-readiness-phase-1-instrument-development.pdf
Graham, C. R., Borup, J., Pulham, E., & Larsen, R. (2018). Blended teaching readiness: Phase 2 – instrument development. Lansing, MI. https://michiganvirtual.org/research/publications/blended-teaching-readiness-phase-2-instrument-development/
Graham, C. R., Borup, J., Pulham, E. B., & Larsen, R. (2019). K–12 blended teaching readiness: Model and instrument development. Journal of Research on Technology in Education, 51(3), 239–258. https://doi.org/10.1080/15391523.2019.1586601
Graham, C. R., Borup, J., Short, C., & Archambault, L. (2019). K–12 blended teaching: A guide to personalized learning and online integration. EdTech Books. https://edtechbooks.org/k12blended
Graham, C. R., Henrie, C. R., & Gibbons, A. S. (2013). Developing models and theory for blended learning research. In A. G. Picciano, C. D. Dziuban, & C. R. Graham (Eds.), Blended learning: Research perspectives, volume 2 (pp. 13-33). Routledge.
Hartshorne, R., Baumgartner, E., Kaplan-Rakowski, R., Mouza, C., & Ferdig, R. E. (2020). Special issue editorial: Preservice and inservice professional development during the COVID-19 pandemic. Journal of Technology and Teacher Education, 28(2), 137–147.
Hanny, C. N., Arnesen, K. T., Guo, Q., Hansen, J., & Graham, C. R. (2021). Barriers and enablers to K-12 blended teaching. Journal of Research on Technology in Education, 1–22. https://doi.org/10.1080/15391523.2021.1991865
Horn, M. B., & Staker, H. (2017). Blended: Using disruptive innovation to improve schools. John Wiley & Sons.
Iyengar, S. S., & Lepper, M. R. (2000). When choice is demotivating: Can one desire too much of a good thing? Journal of Personality and Social Psychology, 79(6), 995–1006. https://doi.org/10.1037/0022-3514.79.6.995
KnowledgeWorks. (2019). What is personalized learning? What is personalized, competency-based learning? Accessed on September 23, 2022. https://knowledgeworks.org/get-inspired/personalized-learning-101/what-personalized-learning/
LEAP Innovations. (2021). The LEAP Learning Framework is the foundation for all of our work. Accessed on March 4, 2021. https://leapinnovations.org/leap-learning-framework/
Moore, M. G. (1993). Theory of transactional distance. Theoretical principles of distance education, 1, 22-38.
Morin, A. (2022). Personalized learning: What you need to know. Accessed on September 23, 2022. https://www.understood.org/en/school-learning/partnering-with-childs-school/instructional-strategies/personalized-learning-what-you-need-to-know
National Center for Learning Disabilities. (2017, June 11). Personalized learning and students with disabilities. Accessed on September 23, 2022. https://www.ncld.org/research/personalized-learning/
Patrick, S., Kennedy, K., & Powell, A. (2013). Mean what you say: Defining and integrating personalized, blended and competency education. International Association for K-12 Online Learning.
Pokhrel, S., & Chhetri, R. (2021). A literature review on impact of COVID-19 pandemic on teaching and learning. Higher Education for the Future, 8(1), 133–141. https://doi.org/10.1177/2347631120983481
Pulham, E., & Graham, C. R. (2018). Comparing K–12 online and blended teaching competencies: A literature review. Distance Education, 39(3), 411–432. https://doi.org/10.1080/01587919.2018.1476840
Pulham, E. B., Graham, C. R., & Short, C. R. (2018). Generic vs. modality-specific competencies for K-12 online and blended teaching. Journal of Online Learning Research, 4(1), 33–52.
Schneider, S., Nebel, S., Meyer, S., & Rey, G. D. (2022). The interdependency of perceived task difficulty and the choice effect when learning with multimedia materials. Journal of Educational Psychology, 114(3), 443–461. https://doi.org/10.1037/edu0000686
Schwartz, B. (2004). The paradox of choice: Why more is less. Ecco.
Schwartz, B., & Ward, A. (2004). Doing better but feeling worse: The paradox of choice. Positive Psychology in Practice, 86-104.
Shemshack, A., & Spector, J. (2020). A systematic literature review of personalized learning terms. Smart Learning Environments, 7. https://doi.org/10.1186/s40561-020-00140-9
Short, C. R., & Graham, C. R. (in review). Blended and personalized: A cross-disciplinary analysis of K–12 blended teaching practices for personalization.
United States Department of Education. (2010). Transforming American education: Learning powered by technology. Office of Educational Technology, Washington, D.C. Accessed on September 23, 2022. http://www.ed.gov/sites/default/files/netp2010.pdf
United States Department of Education. (2017). Reimagining the role of technology in education: 2017 national education technology plan update. Office of Educational Technology, Washington, D.C. Accessed on September 23, 2022. https://tech.ed.gov/files/2017/01/NETP17.pdf
Waterschoot, J., Vansteenkiste, M., & Soenens, B. (2019). The effects of experimentally induced choice on elementary school children’s intrinsic motivation: The moderating role of indecisiveness and teacher–student relatedness. Journal of Experimental Child Psychology, 188, 104692.
Wells, A. S. (2020). Racial, ethnic, and cultural diversity across K–12 and higher education sectors: Challenges and opportunities for cross-sector learning. Change: The Magazine of Higher Learning, 52(2), 56-61. DOI: 10.1080/00091383.2020.1732787